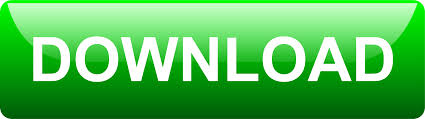
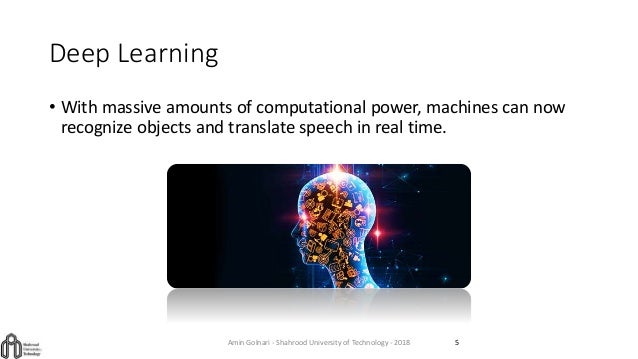
Now, we can discuss the importance of how many GPUs to use for deep learning. In this case, we recommend examining data center GPUs. With all of this in mind, we highly recommend starting out with high-quality consumer-grade GPUs unless you know you are going to be building or upgrading a large-scale deep learning workstation.
Benchmark cpu gpu neural network training full#
These will be far above anything one might do on their own budget or utilize to its full potential on their own. These move beyond simply hobby projects, small business type of projects, and into the realm of corporation-level usage. Systems are plug-and-play, and they may be deployed on bare metal or in containers. Machine learning and deep learning procedures are the focus of these systems. Managed workstations and servers are full-stack, enterprise-grade systems. These GPUs are built for large-scale projects and deliver enterprise-level performance. Truly, these are the best GPUs for deep learning right now. As you begin to get into data points in the billions, though, these types of GPUs will begin to fall off in efficiency and use of time.ĭata center GPUs are the industry standard for deep learning workstations in production.
Benchmark cpu gpu neural network training upgrade#
These GPUs can be used to cheaply upgrade or build workstations and are excellent for model development and low-level testing. Broadly, though, there are three main categories of GPUs you can choose from: consumer-grade GPUs, data center GPUs, and managed workstations, or servers, among these possibilities.Ĭonsumer-grade GPUs are smaller and cheaper, but aren’t quite up to the task of handling large-scale deep learning projects, although they can serve as a starting point for workstations. As we will discuss later on, NVIDIA dominates the market for GPUs, especially for their uses in deep learning and neural networks. That being said, there is a wide range of GPUs to choose from for your deep learning workstation. Most people in the AI community recommend GPUs for deep learning instead of CPUs for this very reason. Obviously, this means you can get more done and done faster by utilizing GPUs instead of CPUs. GPUs can process multiple processes simultaneously whereas CPUs tackle processes in order one at a time. In brief, CPUs are probably the simplest and easiest solution for deep learning, but the results vary on the efficiency of CPUs when compared to GPUs. When you are diving into the world of deep learning, there are two choices for how your neural network models will process information: by utilizing the processing power of CPUs or by using GPUs. Let’s discuss whether GPUs are a good choice for a deep learning workstation, how many GPUs are really needed for deep learning, and which GPUs are the best picks for your deep learning workstation.Īccelerate your machine learning research with an Exxact solution starting around $5,500 There are also two companies who own the GPU market: NVIDIA and AMD. At the end of this guide, we’ll give our best recommendations that excel in each of these areas. When it comes to GPU selection, you want to pay close attention to three areas: high performance, memory, and cooling. The GPU you choose is perhaps going to be the most important decision you'll make for your deep learning workstation. If you are building or upgrading your own deep learning workstation, then you will inevitably begin to wonder, how many GPUs you would need for an AI workstation focused on deep learning or machine learning. Is one adequate, or should you add 2 or 4? Choosing the Right Number of GPUs for a Deep Learning Workstation
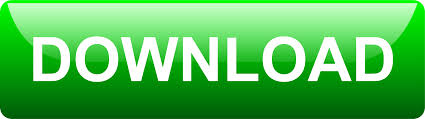